Abstract
With the development of Synthetic Aperture Radar (SAR) imaging and deep learning, the use of deep
learning to classify land cover in SAR images has received extensive attention and applied research. In
this paper, a high-resolution airborne multi-dimensional SAR land cover classification dataset is
constructed based on the high-resolution airborne data of the Chinese Aeronautic Remote Sensing System
(CARSS) for Earth observation, whose name is AIR-MDSAR-Map (Airborne Multi-Dimensional Synthetic
Aperture Radar Mapping Dataset).
The original data are obtained by CARSS, and the platform is a modified Xinzhou 60 remote sensing
aircraft. SAR images and optical images are generated according to the standard data production process.
AIR-MDSAR-Map contains polarization SAR images in bands of C, Ka, L, P, and S and high-resolution
optical images in Wanning, Hainan, and Sheyang, Jiangsu, with the spatial resolution ranging from 0.2 m
to 1 m depending on the band. It divides the land cover into nine categories and generates fine
pixel-level labels through a semi-automatic labeling algorithm. In this paper, the classical semantic
segmentation methods in deep learning, such as UNet, SegNet, Deeplab, and HRNet, are used to verify the
classification of AIR-MDSAR-Map. At the same time, we also test the classification sensitivity of
different band images to all kinds of land cover objects.
This dataset includes multi-dimensional SAR images of the same place and time, which can be used for
fusion classification research. In this paper, multi-dimensional SAR data are fused and classified
through different fusion strategies, model fusion classifies land cover by selectively fusing the models
of each band, and the priori fusion uses the prior information of the classification results in each
band to distinguish land cover on defining the priority of objects. These two fusion methods outperform
the single-band in the performance of some types of land cover, and improve the FWIoU and PA by 10~15%,
the FWIoU reaches 69%, and PA is 81%.
AIR-MDSAR-Map can satisfy the research and application requirements of different users and can be used
to study the characteristics of the same land cover object with different resolutions, bands, and
polarizations. Moreover, it can provide a strong promotion for the development of multi-dimensional SAR
applications. The AIR-MDSAR-Map and our algorithm are available at
github.com/NairongZheng/AIR-MDSAR-Map.
Citation
@article{,
title={Airborne Multi-Dimensional SAR Land Cover Dataset and Fusion Classification Method},
author={Nai-Rong Zheng, Zi-An Yang, Xian-Zheng Shi, Hong Yang, Yue Sun, Feng Wang},
journal={National Remote Sensing Bulletin},
year={2023}
}
AIR-MDSAR-Map
AIR-MDSAR-Map contains polarization SAR images in bands of P, L, S, C, and Ka and high-resolution optical
images in Wanning, Hainan, and Sheyang, Jiangsu. Land cover objects are divided into water, bare soil,
road, industry, vegetation, residence, plantation, farms, and other. Each class is labeled with
different colors, i.e. water in the blue, vegetation in green.
The advantages of the dataset include:
(1) Accurate masks of 9 categories.
(2) Including SAR images of different bands and optical images at the same place and time period.
(3) Difference in features of the same category in the same or different patch images.
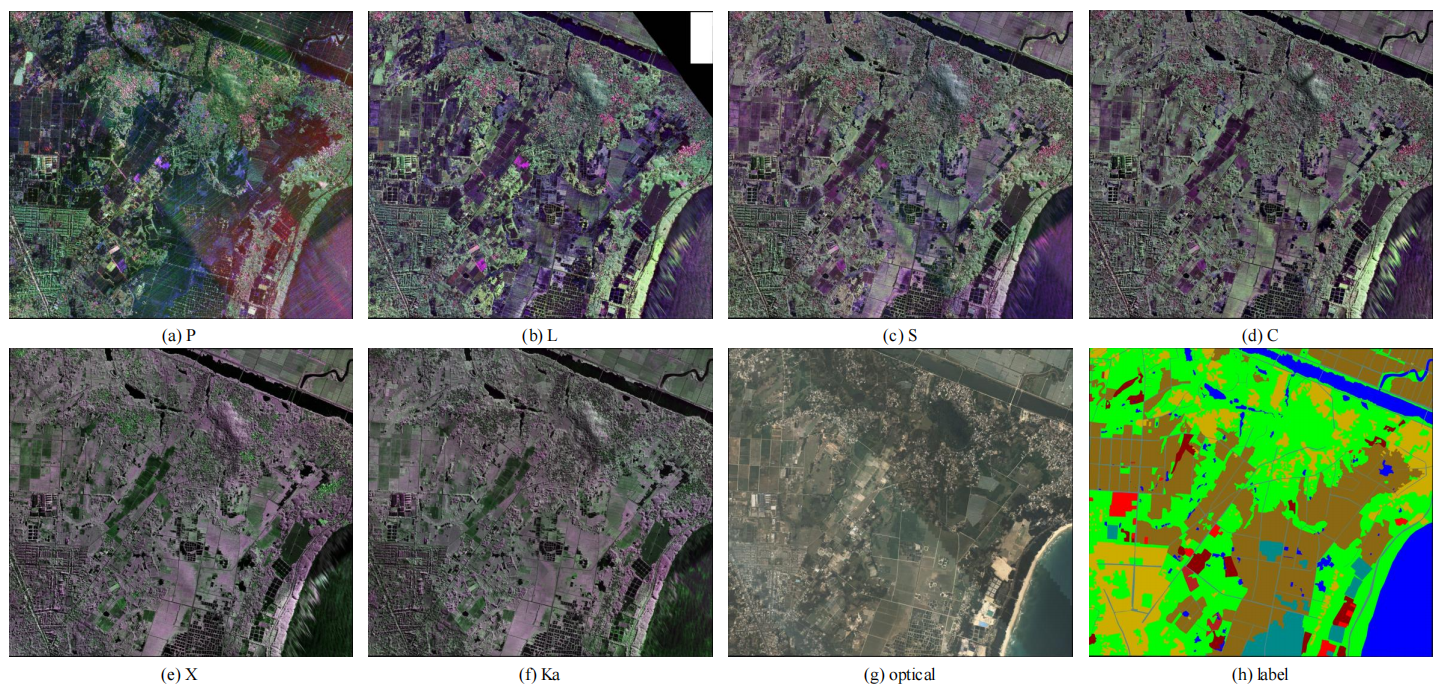
Information of polSAR images used to generate AIR-MDSAR-Map:
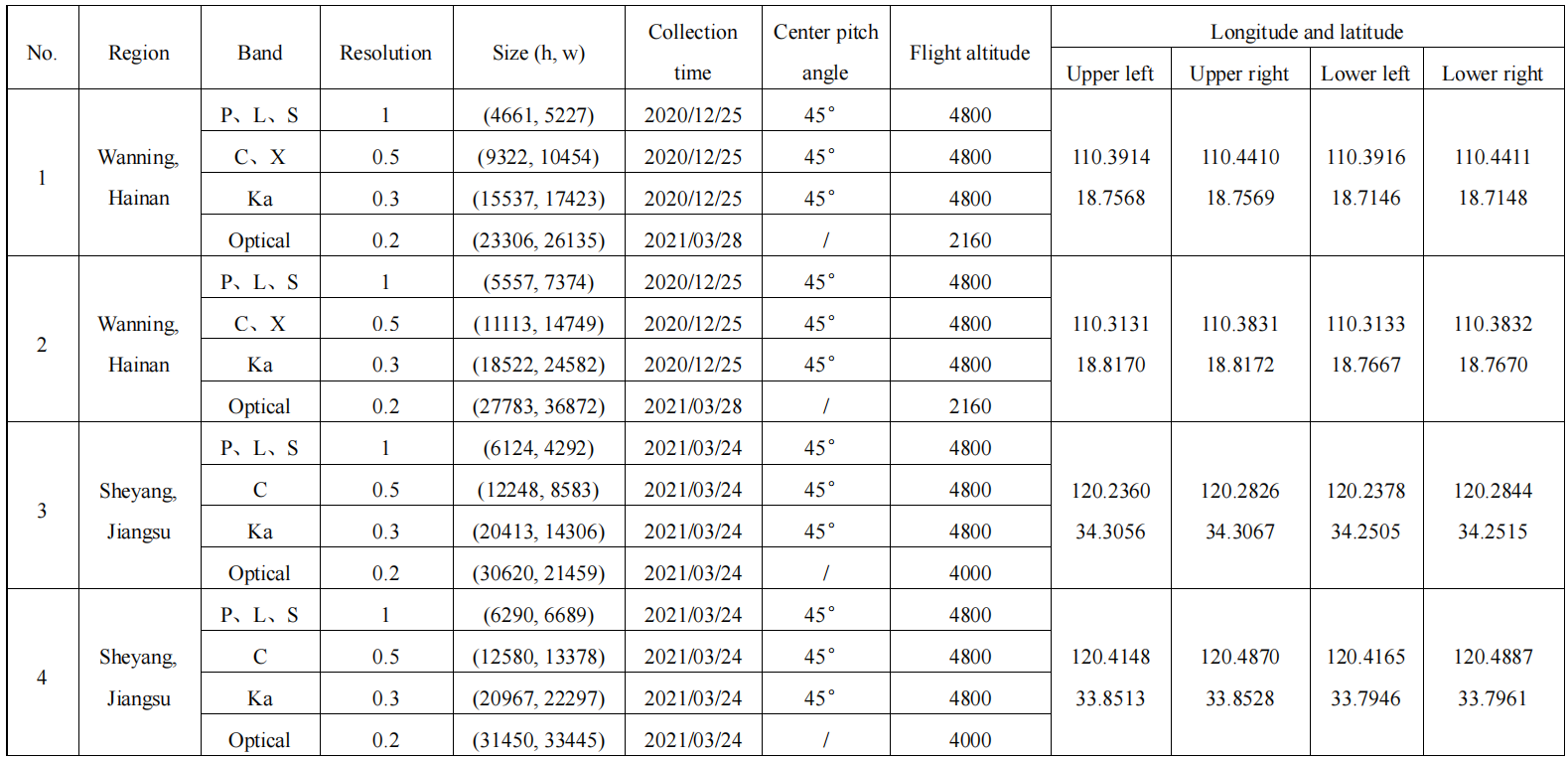
AIR-MDSAR-Map can be downloaded from huggingface:
the raw data of AIR-MDSAR-MapContact
E-mail: fengwang@fudan.edu.cn、nrzheng20@fudan.edu.cn